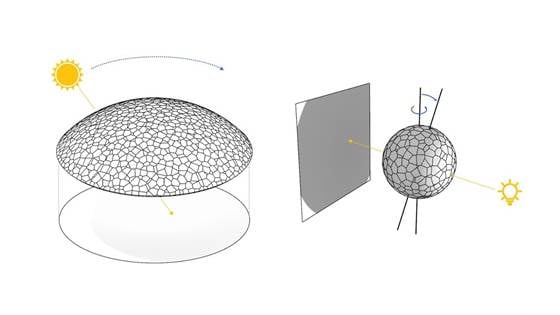
Advanced Shadow Control through Inverse Volume Reconstruction
This project aims to develop the methodology for controlling the cast shadow of an object, by computing the internal structure of the object, and using novel manufacturing techniques like 3D printing to realize it.