Triangulating Outputs of Different Large Language Models for Trustworthy AI
In social sciences, triangulation is a methodology used to validate the reliability of results by cross-verifying them from multiple sources. This thesis proposes a novel approach by applying the idea of triangulation to the outputs of multiple Large Language Models (LLMs) to enhance the trustworthiness of AI.
Contact persons
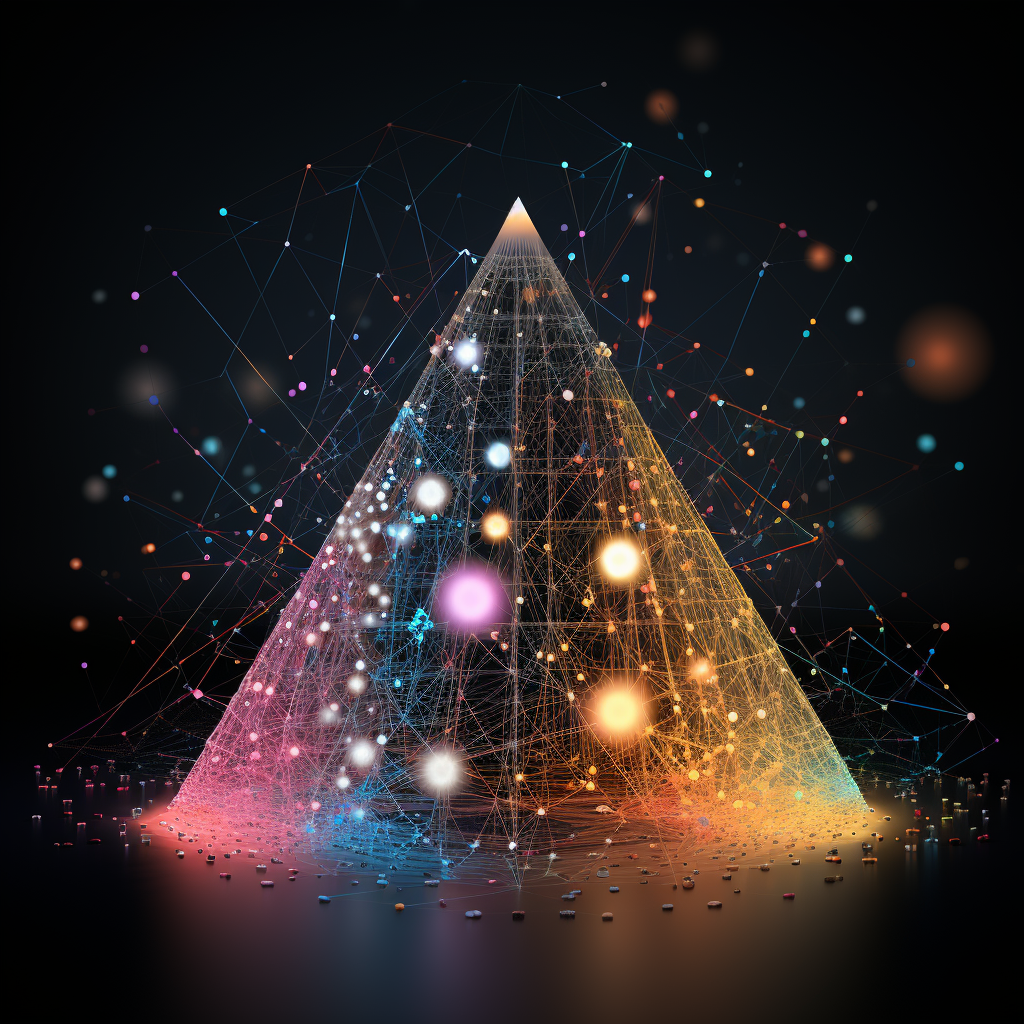
The application area of interest is the identification of security debts in source code, which are potential security risks that may lead to vulnerabilities if left unaddressed.
Research Topic Focus
- Investigate the viability of using multiple LLMs to analyze source code for security debts.
- Develop an algorithm or framework for triangulating the outputs from multiple LLMs.
- Evaluate the accuracy and trustworthiness of triangulated AI outputs compared to individual LLM outputs.
- Understand the trade-offs and implications of using triangulated AI methods for security audits.
Expected Results
- A comparative analysis of the accuracy of individual LLMs versus the triangulated model in identifying security debts.
- A framework or algorithm suitable for integrating the outputs of multiple LLMs.
- Insights into the strengths and weaknesses of individual LLMs in detecting security debts and how triangulation can mitigate these.
Learning Outcomes
- Understand the principles and methods of triangulation in the context of AI and LLMs.
- Develop proficiency in integrating outputs from multiple AI models.
- Gain a deeper understanding of security debts in source code and the intricacies of their detection using AI.
- Acquire skills in evaluating and ensuring the trustworthiness of AI tools in the context of security applications.
Qualifications
- Strong foundation in AI and machine learning principles.
- Familiarity with multiple Large Language Models and their architectures.
- Proficient in programming (preferably Python) and experience with source code analysis.
- Basic understanding of software security principles.
References
- Rindell, Kalle, Karin Bernsmed, and Martin Gilje Jaatun. "Managing security in software: Or: How i learned to stop worrying and manage the security technical debt." Proceedings of the 14th International Conference on Availability, Reliability and Security. 2019.
- Triangulation, Data Source. "The use of triangulation in qualitative research." Oncol Nurs Forum. Vol. 41. No. 5. 2014.
- Patil, Shishir G., et al. "Gorilla: Large language model connected with massive apis." arXiv preprint arXiv:2305.15334 (2023).
- Jain, Shashank Mohan. "Hugging face." Introduction to Transformers for NLP: With the Hugging Face Library and Models to Solve Problems. Berkeley, CA: Apress, 2022. 51-67.
Contact persons/supervisors
Sagar Sen ( Arda Goknil (), Erik Johannes Husom (