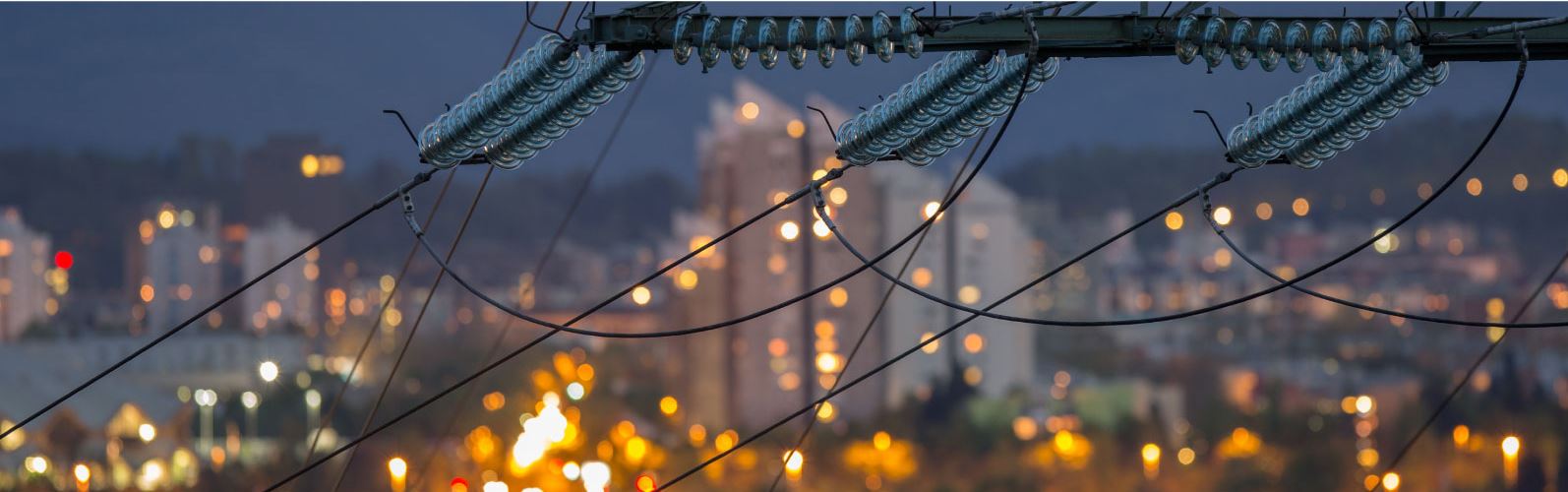
ENERGYTICS
Detecting, classifying and clustering fault signatures
Requirements for quality of electricity supply are specified in the Norwegian regulation of quality of supply[1], and include voltage quality and reliability of supply. In recent years there has been a considerable increase in instrumentation and surveillance of the power system, both through voltage quality meters (PQA), phasor measurement units (PMU), smart electricity meters (AMI), and other types of sensors. The combination of large amounts of data (Big Data) and statistical analysis (machine learning) enables improved handling of faults. Using statistical methods on high resolution data from the electricity grid to predict if a fault situation is developing can give an early warning so that the situation can be avoided and corrected without the consumers being influenced. Overviews and analyses of historical faults will be helpful for the grid operators to report to the authorities, examine causes for faults and possible actions (for example implementing necessary switching operations and disconnection of flexible loads).
This demonstrator is in cooperation with the project EarlyWarn[2], and the tasks most relevant for ENERGYTICS within this demonstrator is to detect periodic faults and events (i.e. cyclic faults and events that occur with strict time intervals between each fault/event), and also to perform clustering of fault events and then classify them into fault categories, to finally be able to determine the underlying cause for the event.