Generative AI to Enable Multi-Agent Conversations
Contact persons
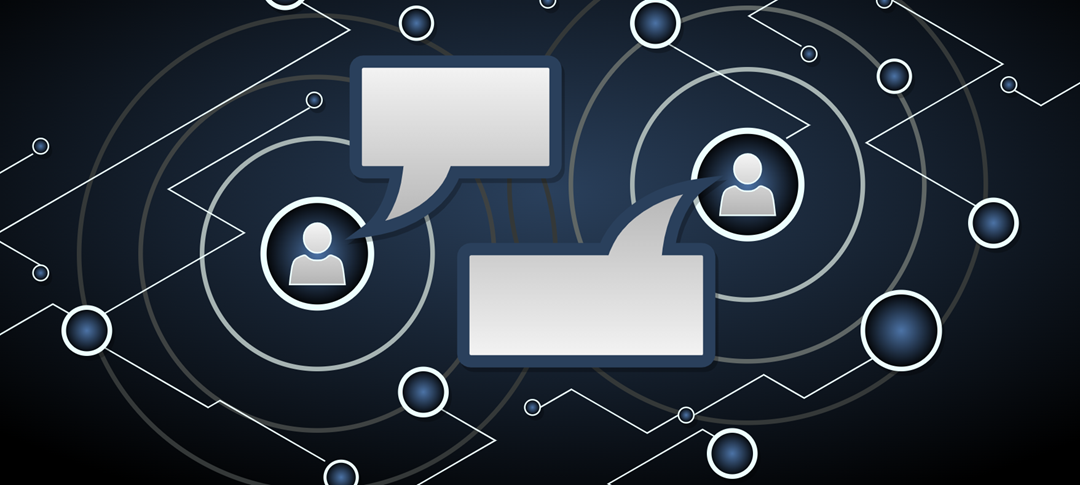
Master Thesis Description
In the digital world, interactions are often characterized by predefined workflows and lack of semantic comprehension. Multi-agent conversations can improve or replace these interactions, allowing systems to collaborate in a human-like manner. The thesis objective is to enhance interoperability by implementing the actor model, exploring the interaction dynamics among multiple agents (or actors), and addressing their limitations.
Research Topic Focus
- Analysing the state-of-the-art in multi-agent conversation systems.
- Developing a framework that adheres to the actor model guidelines, facilitating effective communication and coordination between agents.
- Use of open source LLMs as part of agent creation.
- Implementing and testing the framework within simulated or real edge-cloud continuum environments.
Expected Results
- A comprehensive analysis of the challenges and opportunities in current system communication and multi-agent conversation systems.
- A novel multi-agent framework aligned with the actor model principles, enabling efficient and reliable collaboration between LLM-based agents.
- Demonstrated improvements in the collaborative efficiency of multi-agent conversations compared to state-of-the-art.
- Insights into the applicability of the proposed solutions across various use cases.
Learning Outcomes
- Acquire hands-on experience in designing and implementing advanced conversational agents and interaction strategies.
- Gain a better understanding of the technological underpinnings of generative AI, LMMs and multi-agent conversation systems.
- Improve ability to critically assess the performance and effectiveness of generative AI-enhanced systems.
- Innovate the field of AI.
Qualifications
- Strong background in machine learning, AI and LLMs.
- Proficiency in programming languages commonly used in AI development, with a preference for Python.
- Analytical and creative thinking.
References
Wu, Q., Bansal, G., Zhang, J., Wu, Y., Zhang, S., Zhu, E., ... & Wang, C. (2023). Autogen: Enabling next-gen LLM applications via multi-agent conversation framework. arXiv preprint arXiv:2308.08155.
Josifoski, M., Klein, L., Peyrard, M., Li, Y., Geng, S., Schnitzler, J. P., ... & West, R. (2023). Flows: Building blocks of reasoning and collaborating AI. arXiv preprint arXiv:2308.01285.
Hewitt, C. (2010). Actor model of computation: scalable robust information systems. arXiv preprint arXiv:1008.1459.