Controlled surrogate models in industrial settings
Contact persons
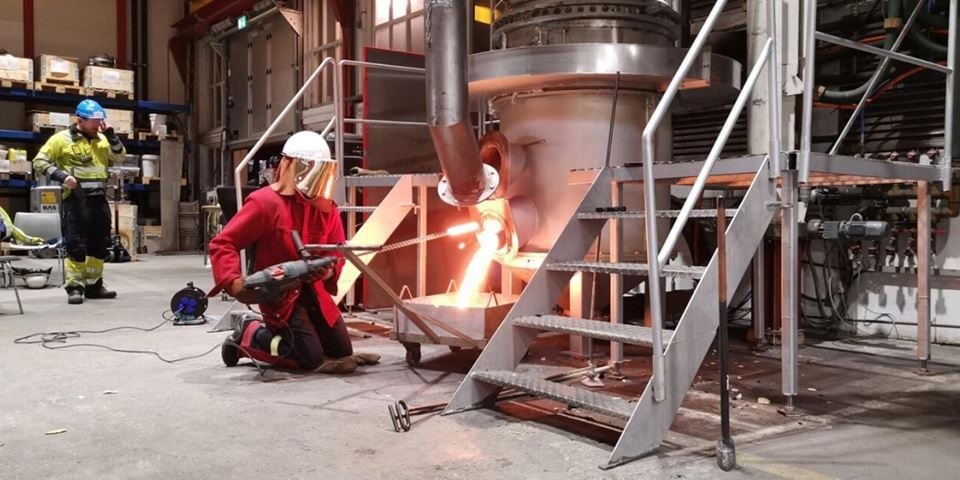
SINTEF collaborates with industry partners on how to use machine learning to improve quality and efficiency of industrial production processes.
For instance, in simulations of such processes, accounting for all the physics involved quickly leads to large and computationally intensive models, which makes real-time predictions difficult. To handle this, we can use surrogate models, which are simplified approximations of more complex models. These map input data to output data when the dependence between the two is unknown or computationally expensive to evaluate.
For instance, in the case of an industrial furnace, this should be kept at specific temperatures throughout the production process to assure the product quality. It is then necessary to control the heating process to reach and keep the temperature at the desired values.
Projects
To make surrogate models more relevant for industry applications, a master project could address the following:
- Develop machine learning-based surrogate models which incorporate control.
- Study and try to quantify how control input affects the quality of model predictions.