Predicting peak load in secondary substations
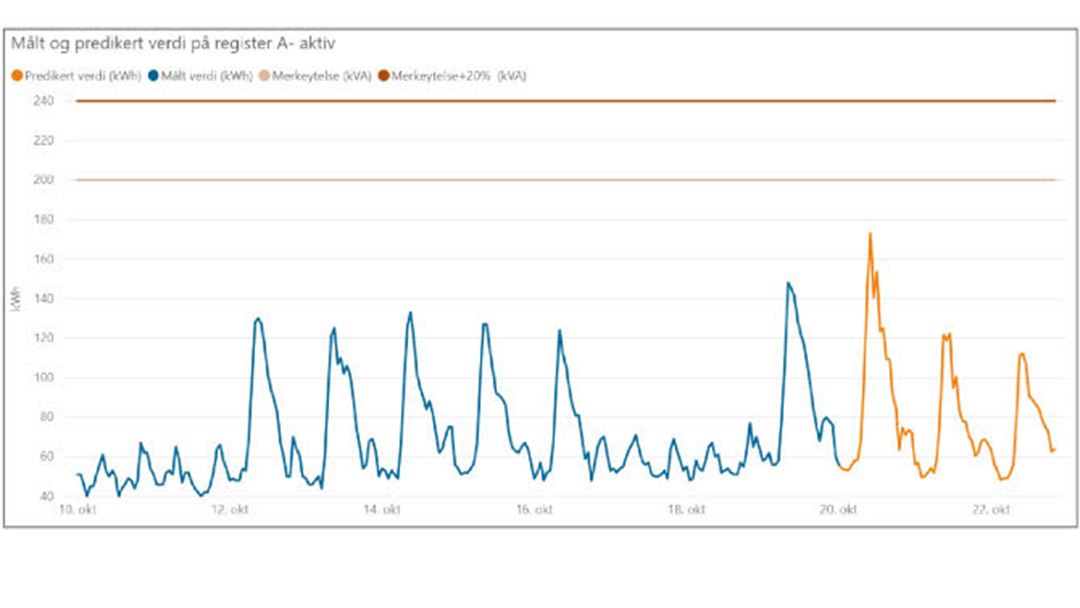
Secondary substations can get overloaded in peak load situations. This may lead to need for maintenance, reduced lifetime and outages.
Load forecasting can be used to detect overload, and then possibly to take actions to avoid overload or mitigate the consequences. This may be done e.g. by using demand response, operational readiness, repair, or upgrade the secondary substation.
Together with Glitre Nett, CINELDI is looking to use machine learning to forecast loads on secondary substations.
In the pilot we have used hourly load from 26 substations and weather data from Norwegian Meteorological Institute as input data. Different machine learning methods were used and compared to baseline methods using MAPE (Mean Absolute Percentage Error).
There was no «one model best in all cases», but LGBM with individual models (i.e. model trained individually for each substation load profile) emerges as the best option. SARIMAX and the naive models performed well in cases where the load is repetitive.
A fully automated machine learning pipeline is in production at Glitre Nett. It is currently predicting load for 123 secondary substations, with a model based on XGBoost. The model is retrained regularly.
Publications:
- Pilot "Predicting peak load in secondary substations" report (in Norwegian)