Robust Model Predictive Control in Energy Systems: Dealing with Uncertainty
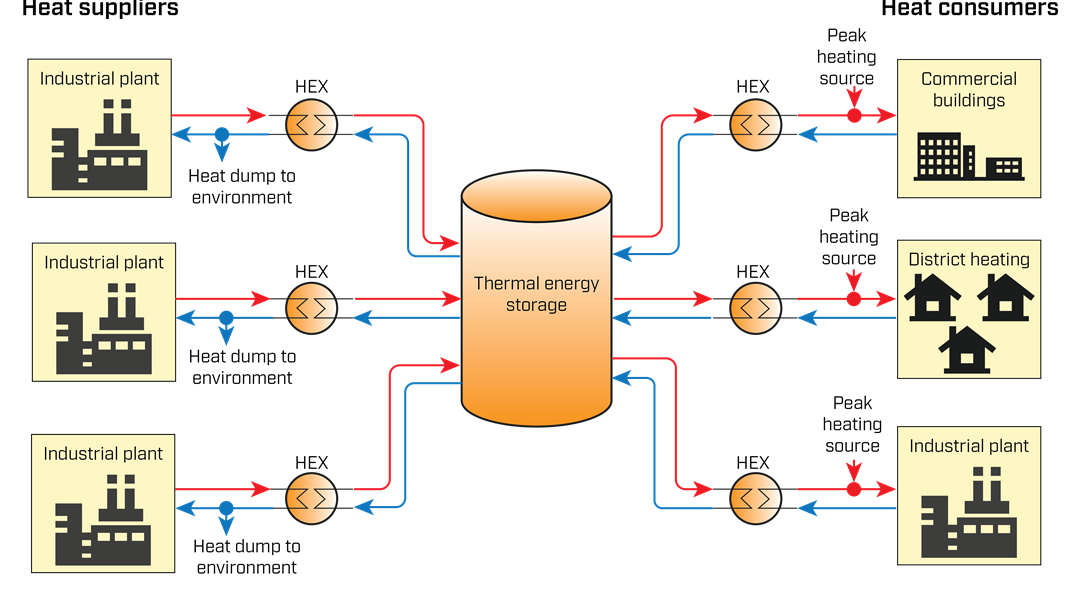
Mandar Thombre, PhD, NTNU
Model predictive control (MPC) is a powerful tool that has been widely used for optimisation in the chemical process industry. A key challenge in such process optimisation is that a large majority of real-world processes lack perfect system information and must contend with significant uncertainties. An example of this is industrial waste heat recovery, where daily operation involves heat supply and demand varying significantly from their predicted profiles.
To counter this uncertainty, my PhD project investigated a framework of “robust” MPC where the uncertainty is described in form of a scenario tree, with the scenarios representing a unique combination of uncertain parameters. We proposed novel algorithms within this framework that focused on two important aspects: 1) selecting scenarios that better describe the uncertainty, and 2) improving its computational efficiency for solving large problems. The new framework was applied to benchmark problems as well as an industrial case-study focusing on thermal energy storage in district heating.